Fraud Detection Using Big Data Analytics
Learn how big data analytics transforms fraud detection with advanced techniques like machine learning and real-time monitoring. Enhance your strategy today!
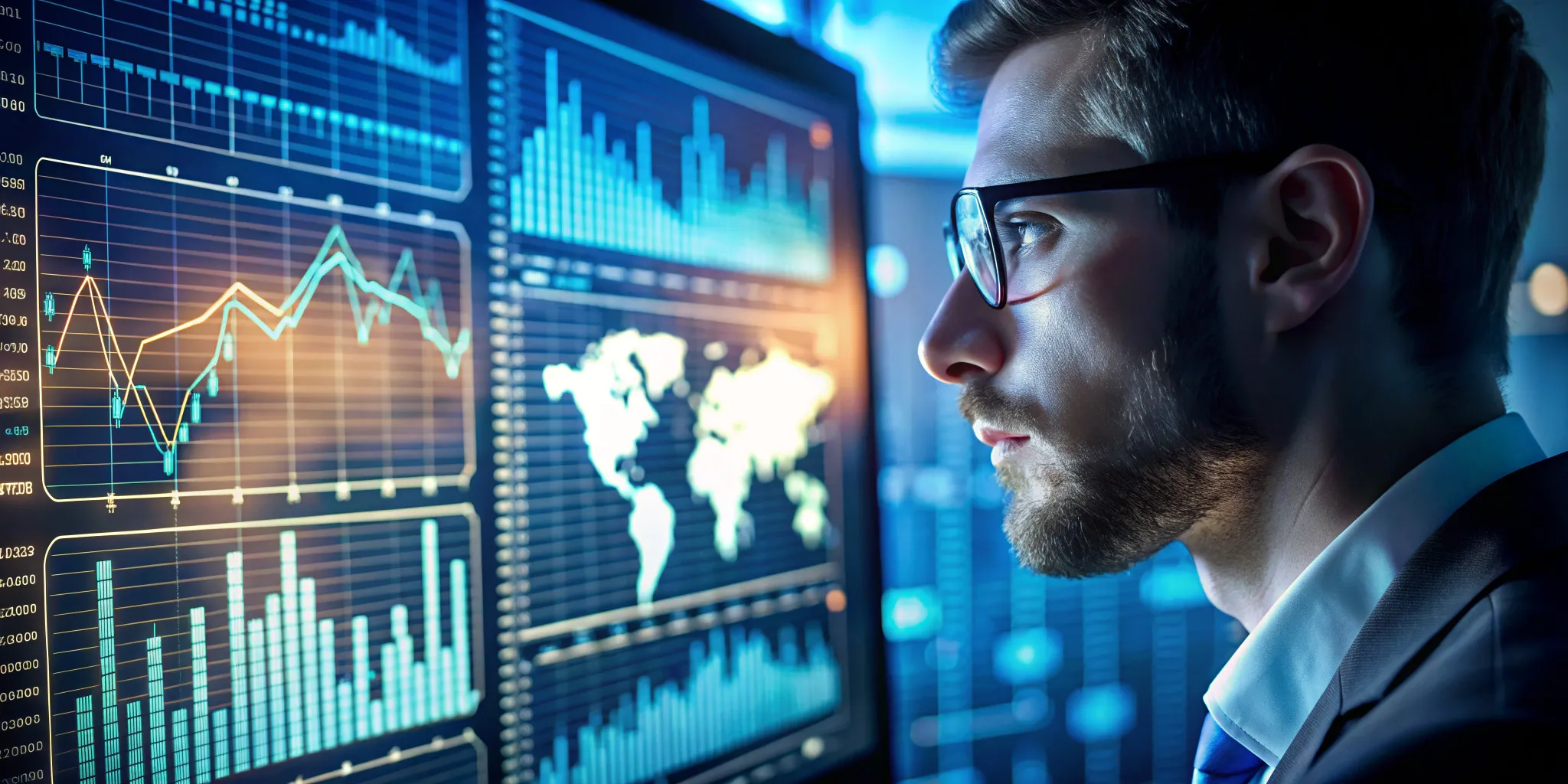
Fraudsters are getting smarter, and traditional detection methods just aren’t cutting it anymore. Enter big data analytics—a game-changer for businesses looking to stay one step ahead. This powerful tool sifts through mountains of data to spot patterns and anomalies that hint at fraudulent activities. Ready to understand how big data can transform your fraud detection strategy? Let’s break it down.
Key Takeaways
Big Data Analytics Enhances Fraud Detection: By analyzing large datasets, businesses can identify patterns and anomalies that indicate fraudulent activities, making traditional detection methods obsolete.
Advanced Techniques Drive Effectiveness: Utilizing machine learning algorithms, real-time data processing, and predictive analytics significantly improves the accuracy and speed of fraud detection.
Continuous Adaptation is Crucial: Maintaining high-quality data, ensuring continuous monitoring, and leveraging the latest advancements in AI and IoT are essential for staying ahead of evolving fraud tactics.
What is Big Data Analytics in Fraud Detection?
Big data analytics is like a super detective for businesses, constantly scanning through massive amounts of data to catch any sneaky fraudulent activities. It involves analyzing large datasets to detect patterns and anomalies that could indicate fraud. By leveraging big data, companies can spot suspicious behavior early and take action before any damage is done.
Key Features of Big Data Analytics
Real-Time Data Processing: One of the standout features of big data analytics is its ability to process information in real-time. This means businesses can detect and respond to fraudulent activities almost immediately, reducing the risk of significant financial losses.
Machine Learning Algorithms: These algorithms learn from historical data and get better over time at identifying potential fraud. They can recognize subtle patterns that might be missed by traditional methods.
Predictive Analytics: Predictive analytics uses historical data to forecast future events. In fraud detection, this means predicting which transactions are likely to be fraudulent based on past trends.
How Does Big Data Help in Fraud Detection?
Big data analytics helps in fraud detection by providing powerful tools for pattern recognition, anomaly detection, and predictive modeling.
Pattern Recognition
Big data analytics excels at identifying recurring patterns that may indicate fraudulent activities. For example, if a credit card is suddenly used in multiple locations within a short time frame, this could be flagged as suspicious behavior. By recognizing these patterns, businesses can take proactive measures to prevent fraud.
Anomaly Detection
Anomaly detection involves spotting deviations from normal behavior that could suggest fraud. For instance, if an account suddenly makes an unusually large transaction or logs in from an unfamiliar location, these anomalies can trigger alerts for further investigation.
Predictive Modeling
Predictive models use historical data to forecast future fraudulent activities. By analyzing past incidents of fraud, these models can identify characteristics and behaviors commonly associated with fraudulent transactions. This allows businesses to predict and prevent potential fraud before it happens.
Techniques Used in Fraud Detection
Several analytical techniques are employed in fraud detection using big data analytics:
Logistic Regression
Logistic regression helps predict the probability of a transaction being fraudulent by analyzing various factors such as transaction amount, location, and frequency. It assigns a probability score to each transaction, indicating how likely it is to be fraudulent.
Decision Trees
Decision trees classify transactions into different categories based on certain criteria. They help identify which transactions are most likely to be fraudulent by following a series of decision rules derived from historical data.
Neural Networks
Neural networks are advanced algorithms capable of identifying complex patterns in large datasets. They mimic the human brain's ability to learn from experience and improve over time. In fraud detection, neural networks can uncover hidden relationships between different variables that may indicate fraudulent behavior.
By leveraging these techniques and the power of big data analytics, businesses can significantly enhance their ability to detect and prevent fraud effectively.
Note: The links provided here serve as placeholders for where you might naturally include hyperlinks when they become available or relevant sources are found.
Real-World Examples of Fraud Detection Using Big Data Analytics
Big data analytics is revolutionizing how various industries detect and prevent fraud. Let's dive into some practical examples of how different sectors are leveraging this technology.
Financial Services
Banks and financial institutions are at the forefront of using big data analytics to combat fraud. By analyzing vast amounts of transaction data, they can identify suspicious activities in real-time. For instance, banks use machine learning algorithms to detect unusual patterns in spending behavior, such as a sudden spike in transactions or purchases from atypical locations. This proactive approach allows them to flag potentially fraudulent activities before significant losses occur.
One notable example is HSBC, which has implemented advanced analytics and AI to enhance its fraud detection capabilities. The bank's system continuously monitors transactions and uses predictive models to identify potential fraud, significantly reducing false positives and improving the accuracy of detections.
E-commerce
Online retailers face unique challenges when it comes to fraud detection, given the high volume of transactions and the speed at which they occur. Big data analytics enables e-commerce companies to monitor transactions in real-time, spotting anomalies that could indicate fraudulent activity.
For example, Amazon employs sophisticated machine learning models that analyze customer behavior, purchase history, and other relevant data points to detect unusual patterns. If a transaction deviates from the norm—such as an unusually large order or multiple purchases from different geographic locations—the system flags it for further review.
Telecommunications
Telecom companies also leverage big data analytics to prevent fraud, particularly through predictive analytics. By analyzing call detail records (CDRs) and customer usage patterns, telecom providers can identify irregularities that may suggest fraudulent activities such as SIM card cloning or unauthorized account access.
A case in point is Verizon, which uses predictive models to analyze billions of call records daily. This allows them to detect anomalies quickly and take preventive measures before substantial damage occurs.
Challenges in Implementing Big Data Analytics for Fraud Detection
While big data analytics offers powerful tools for detecting fraud, implementing these solutions comes with its own set of challenges.
Data Quality
High-quality data is crucial for effective fraud detection. Inaccurate or incomplete data can lead to false positives or missed fraud attempts. Ensuring data quality involves rigorous processes for data collection, cleansing, and validation.
Organizations often struggle with integrating disparate data sources into a cohesive system. For instance, combining transaction records from multiple platforms requires consistent formatting and accurate timestamping—any discrepancies can compromise the effectiveness of the analytics.
Resource Constraints
Implementing big data analytics requires significant resources, including advanced software tools and skilled personnel capable of managing complex datasets and algorithms. Smaller businesses may find it challenging to allocate sufficient budget and expertise toward these initiatives.
Moreover, maintaining an up-to-date infrastructure that supports real-time processing can be costly. Continuous investment in technology upgrades is necessary to keep pace with evolving fraud tactics but may strain limited resources.
Best Practices for Using Big Data Analytics in Fraud Detection
To maximize the effectiveness of big data analytics in detecting fraud, businesses should adhere to several best practices:
Ensuring Data Quality
Maintaining high-quality data starts with robust collection methods—ensure all relevant information is captured accurately at the point of entry. Regular audits and automated validation checks help identify inconsistencies early on.
Investing in tools that facilitate seamless integration across various platforms can also enhance overall data quality. Solutions like ETL (Extract, Transform, Load) processes streamline this integration by automating much of the heavy lifting involved in combining disparate datasets.
Continuous Monitoring
Real-time monitoring is essential for timely detection of fraudulent activities. Implement systems that provide continuous oversight rather than periodic checks—this allows immediate response when anomalies are detected.
Regular updates to your monitoring algorithms ensure they remain effective against new types of fraud attempts as they emerge over time. Staying current with industry trends helps keep your defenses sharp against evolving threats.
Leveraging Machine Learning
Machine learning algorithms are invaluable assets in modern fraud detection strategies—they adapt over time based on new inputted information without requiring manual reprogramming after every update cycle.
Integrating machine learning involves selecting appropriate models tailored specifically toward identifying relevant patterns within your dataset—for example clustering techniques might reveal hidden relationships between seemingly unrelated events indicative potential fraudulent behavior.
Utilize pre-trained models where possible but don’t shy away from customizing them according unique characteristics observed within your own operational context—this personalization often yields better results than generic out-of-the-box solutions alone could provide.
How Edge Can Help with Fraud Detection
Edge is at the forefront of providing innovative solutions for fraud detection. With services like transaction risk scoring and subscription payment management, businesses can significantly enhance their ability to detect and prevent fraudulent activities.
Transaction Risk Scoring
Transaction risk scoring is a powerful tool that helps identify high-risk transactions before they cause any damage. Edge’s solution analyzes various data points to assign a risk score to each transaction. This score indicates the likelihood of the transaction being fraudulent. By leveraging machine learning algorithms and real-time data analysis, Edge ensures that businesses can quickly flag suspicious activities and take preventive measures.
For example, if a transaction exhibits unusual patterns—like an unusually high amount or an out-of-ordinary location—the system assigns a higher risk score, prompting further investigation or automatic blocking of the transaction. This proactive approach not only reduces potential losses but also enhances overall customer trust.
Subscription Payment Management
Managing subscription payments can be a complex task, especially when it comes to preventing fraud. Edge’s subscription payment management system streamlines this process by incorporating robust fraud detection mechanisms. It monitors recurring transactions for anomalies and flags any irregularities that may indicate fraudulent behavior.
This system also ensures that legitimate transactions are processed smoothly, minimizing disruptions for genuine customers. By automating these processes, businesses can focus more on growth and less on manual monitoring tasks.
Future Trends in Fraud Detection Using Big Data Analytics
The landscape of fraud detection is continually evolving, driven by advancements in technology and emerging trends. Here’s what the future holds for fraud detection using big data analytics:
AI and Machine Learning Advancements
Artificial intelligence (AI) and machine learning are revolutionizing fraud detection. These technologies enable systems to learn from past data, adapt to new patterns, and predict future fraudulent activities with greater accuracy. As AI continues to advance, we can expect even more sophisticated models capable of detecting complex fraud schemes that traditional methods might miss.
Increased Integration with IoT
The Internet of Things (IoT) is becoming increasingly integrated into various aspects of business operations. This integration brings new opportunities for enhanced fraud detection through big data analytics. IoT devices generate vast amounts of real-time data that can be analyzed to identify unusual patterns or behaviors indicative of fraud.
For instance, in the financial sector, IoT-enabled devices could monitor physical transactions at ATMs or point-of-sale terminals in real time, providing an additional layer of security against fraudulent activities.
Enhanced Real-Time Capabilities
Real-time data processing is crucial for effective fraud detection. The trend towards enhanced real-time capabilities means that businesses will be able to detect and respond to fraudulent activities almost instantaneously. With big data analytics tools continuously improving their ability to process large datasets quickly, organizations can stay ahead of fraudsters by identifying suspicious activities as they happen.
By staying informed about these trends and adopting cutting-edge technologies like those offered by Edge, businesses can strengthen their defenses against ever-evolving fraud tactics.
Staying Ahead of Fraud with Big Data Analytics
Big data analytics has undoubtedly transformed the landscape of fraud detection, offering businesses a powerful set of tools to identify and prevent fraudulent activities effectively. By leveraging advanced techniques such as machine learning, real-time data processing, and predictive analytics, organizations can stay one step ahead of fraudsters.
However, implementing these solutions isn't without its challenges. Ensuring data quality and managing resource constraints are crucial steps in building an effective fraud detection system. Adhering to best practices—like maintaining high-quality data, continuous monitoring, and integrating machine learning algorithms—can significantly enhance your fraud prevention efforts.
Edge stands out as a key player in this field, offering innovative solutions like transaction risk scoring and subscription payment management to help businesses safeguard against fraud. As we look to the future, advancements in AI and machine learning, increased integration with IoT devices, and enhanced real-time capabilities promise even more robust defenses against evolving fraud tactics.
By embracing these technologies and staying informed about emerging trends, businesses can not only protect themselves from financial losses but also build stronger trust with their customers. Ready to take your fraud detection strategy to the next level? Explore what Edge has to offer and stay ahead of the curve.
Frequently Asked Questions
How does big data analytics help in fraud detection?
Big data analytics helps by analyzing large datasets to identify patterns and anomalies that indicate fraudulent activities. It uses machine learning algorithms, real-time data processing, and predictive analytics to spot suspicious behavior early, allowing businesses to take proactive measures.
What are the key features of big data analytics in fraud detection?
Key features include real-time data processing, which allows immediate detection and response; machine learning algorithms that improve over time by learning from historical data; and predictive analytics that forecast future fraudulent activities based on past trends.
What techniques are used in big data analytics for fraud detection?
Common techniques include logistic regression, which predicts the probability of a transaction being fraudulent; decision trees, which classify transactions based on various criteria; and neural networks, which identify complex patterns indicative of fraud by mimicking the human brain's learning process.
Can you provide real-world examples of industries using big data for fraud detection?
Sure! In financial services, banks like HSBC use advanced analytics and AI to monitor transactions in real-time. E-commerce giants like Amazon employ machine learning models to detect unusual purchase behaviors. Telecom companies such as Verizon utilize predictive models to analyze call records and prevent fraud.
What challenges do businesses face when implementing big data analytics for fraud detection?
Key challenges include ensuring high-quality data, as inaccurate or incomplete information can lead to false positives or missed fraud attempts. Resource constraints are another issue since implementing these solutions requires significant investment in technology and skilled personnel capable of managing complex datasets and algorithms.
Related Articles
Unveiling AI: The Future of Fraud Detection in Payment Processing - Edge
How to Reduce Risk in Payments: Leveraging Machine Learning and AI - Edge
The Ultimate Guide to Mastering Transaction Risk Scoring for Fraud Prevention - Edge
Understanding Why Businesses Need to Prioritize Fraud Protection in Payment Processing - Edge
© 2025 Edge Payment Technologies, Inc.
6600 Sunset Blvd. Ste. 226 Los Angeles, CA. 90028
(323)-388-3931
Registered ISO of FFB Bank and Chesapeake Bank